Artificial intelligence (AI) technologies remain an ongoing trend in software development, with the global AI market forecasted to grow to $309.6 billion by 2026. No wonder cloud service providers (CSPs) are offering AI-driven services for detecting objects in video, recognizing faces of celebrities, and turning speech into text.
Some of these providers have even taken steps toward offering more complex artificial intelligence platform as a service (AI PaaS) solutions. These solutions are designed to help developers build products that use machine learning (ML) and deep learning (DL) faster and with less effort.
In this article, we explore what AI PaaS is and take a look at its key characteristics. We also discuss the pros and cons of using such platforms for software development and look at popular AI PaaS vendors. This article will be helpful for developers and business owners who want to introduce new AI capabilities to their products.
Contents:
Moving from custom AI solutions to cloud-based AI services
Artificial intelligence technologies can be helpful in numerous use cases such as analyzing facial emotions, classifying cancer types, detecting malware, recognizing speech, and enhancing car insurance services.
But since AI is a resource-hungry technology, developing intelligent algorithms from scratch may be costly. Therefore, many organizations use AI as a service (AIaaS) and AI PaaS products from cloud vendors to experiment with AI-based technologies. Let’s explore the main differences between these two services:
Now, let’s take a closer look at each of these services.
Artificial intelligence as a service
AIaaS covers ready-to-use AI products and services (with built-in ML and DL algorithms) for data management with limited customization capabilities. Such software helps organizations with advanced trend analysis, pattern matching, predictive analytics, and other data management tasks. AIaaS services usually are affordable for small and midsize businesses.
There are dedicated AI solutions meant for solving specific tasks like:
- Processing and analyzing big data
- Processing specific types of data (image, video, speech)
- Building prediction models
- And more
Common AIaaS use cases are:
- Chatbots
- Digital assistant solutions
- ML and AI frameworks that help developers build their own models
Today, however, there’s an emerging trend among AI service providers: they’re expanding their offerings to full-scale platforms for AI developers. This is where AI platforms as a service come into play.
Looking for a way to adopt AI quickly?
Let’s find the best way to integrate cloud AI services into your systems and applications.
Artificial intelligence platform as a service
AI PaaS is a set of AI and ML platform services for building, training, and deploying AI-powered application functionalities. Since by definition, PaaS services help users develop, run, and manage applications, AI PaaS can help organizations create AI-based products without the need to purchase and maintain infrastructure.
This term usually refers to end-to-end solutions like cloud platforms that allow businesses to use AI-based services they need on a pay-per-use or pay-per-service basis. To provide comprehensive intelligent solutions that can work out of the box, such platforms often include managed sub-services and third-party APIs.
Major cloud service providers offer both AIaaS and AI PaaS solutions within a single platform. So although these services are different, they are often combined in a single product. In this article, we mostly focus on the AI PaaS model. Now let’s take a look at the key elements an AI PaaS service may include.
AI PaaS key components
First, let’s look at the concept of AI PaaS from the perspective of the classic platform as a service (PaaS) model.
With PaaS, a cloud vendor provides an environment for building, deploying, and maintaining applications. A basic PaaS environment usually includes two main groups of components required for application development: infrastructure and a platform.
Now, let’s go back to AI PaaS. AI itself is all about processing enormous amounts of data, which, in turn, requires extensive computing power. This is why, similar to the standard PaaS model, many AI service providers offer infrastructure resources, computing resources, and virtualization capabilities. All the big data required for training ML models and improving AI solutions needs to be stored somewhere. This is why data storage resources are a common element of AIaaS and AI PaaS products.
What distinguishes AI PaaS from a basic PaaS model are two components: pretrained ML models and AI APIs.
Let’s take a closer look at each of these components.
Read also
Explaining Cloud Computing Models: SaaS, PaaS, and IaaS
Сhoose the model that suits best both your business needs and project requirements. Apriorit’s specialists prepared a comprehensive article comparing use cases, benefits, and challenges of adopting IaaS, PaaS, and SaaS.
1. Pretrained machine learning models
ML algorithms and models play the main role in implementing AI-based solutions: they process and analyze data, solve specific tasks, and provide the end result you expect. However, building and training machine learning and deep learning models from scratch requires enormous resources and expertise.
This is why many cloud vendors offer pretrained models and algorithms that can perform the following specific tasks:
- Extract features
- Make predictions
- Recognize speech
- Run complex calculations
- Classify videos
- Detect moving objects and video
- Process images
- And more
Some pretrained AI services are non-customizable and can only perform a limited set of operations, while others can be customized to the needs of a particular project.
2. AI APIs
Application programming interfaces (APIs) make it even easier to implement AI functionalities in your application. Some vendors provide ready-to-use APIs for various AI functionalities as part of their platforms or as an independent service.
The most common APIs offered by AI service providers include APIs for:
- Computer vision
- Natural language processing
- Text-to-speech conversion
- Computer speech
- Translation
- Emotion detection and other options
Now, let’s examine the benefits and challenges of using AI PaaS services.
Pros and cons of using AI PaaS services
Cloud service providers make AI capabilities available for developers, data scientists, business owners, and researchers. They often claim that their services can help businesses significantly simplify the development process and accelerate a product’s time to market. Let’s take a look at the most important pros and cons of using an AI PaaS solution in your project.
Benefits of using AI PaaS
Using a comprehensive platform for developing AI products helps organizations in various ways.
- Reduced development costs and time. AI PaaS products provide a variety of helpful tools and services that significantly simplify all stages of application development. Thus, developers can build, train, and test ML models faster and have more time to work on other application components. Businesses don’t need to spend time and effort on purchasing and maintaining costly hardware. Plus, the traditional pay-as-you-go model used by most CSPs allows you to effectively control your spending.
- Premade infrastructure and environment. PaaS services usually offer secure environments for developers to work on AI algorithms and build and deploy their solutions. Another bonus is that modern AI PaaS solutions support the most popular AI frameworks, libraries, tools, and programming languages.
- High scalability. You can start small and scale your AI-based project as your needs grow, without worrying about computational power. Scalability can be crucial when processing huge sets of data, deploying your solution across multiple platforms, and so on.
- Built-in tools for working with datasets. Designed specifically to help developers build AI solutions, AI PaaS products often provide tools that help to overcome common challenges. And one of the most common pitfalls in AI development is ensuring high-quality datasets. Some platforms, for instance, provide efficient tools for labeling data, detecting poor quality data, and detecting bias.
- Ready-to-go AI algorithms. AI PaaS providers usually offer their own algorithms trained to solve common tasks like detecting objects, text, and emotions. Developers can use these ready-to-go algorithms in their products as they are or customize them according to their software’s needs.
Now, let’s overview the key drawbacks of using third-party AI services in your projects.
Related project
Leveraging NLP in Medicine with Custom Model for Scientific Reports Analysis
Explore how Apriorit’s AI developers helped our client improve research capabilities and speed up data analysis. Uncover the details of enabling a medical research client to extract critical information from clinical text, accelerating their scientific research process.
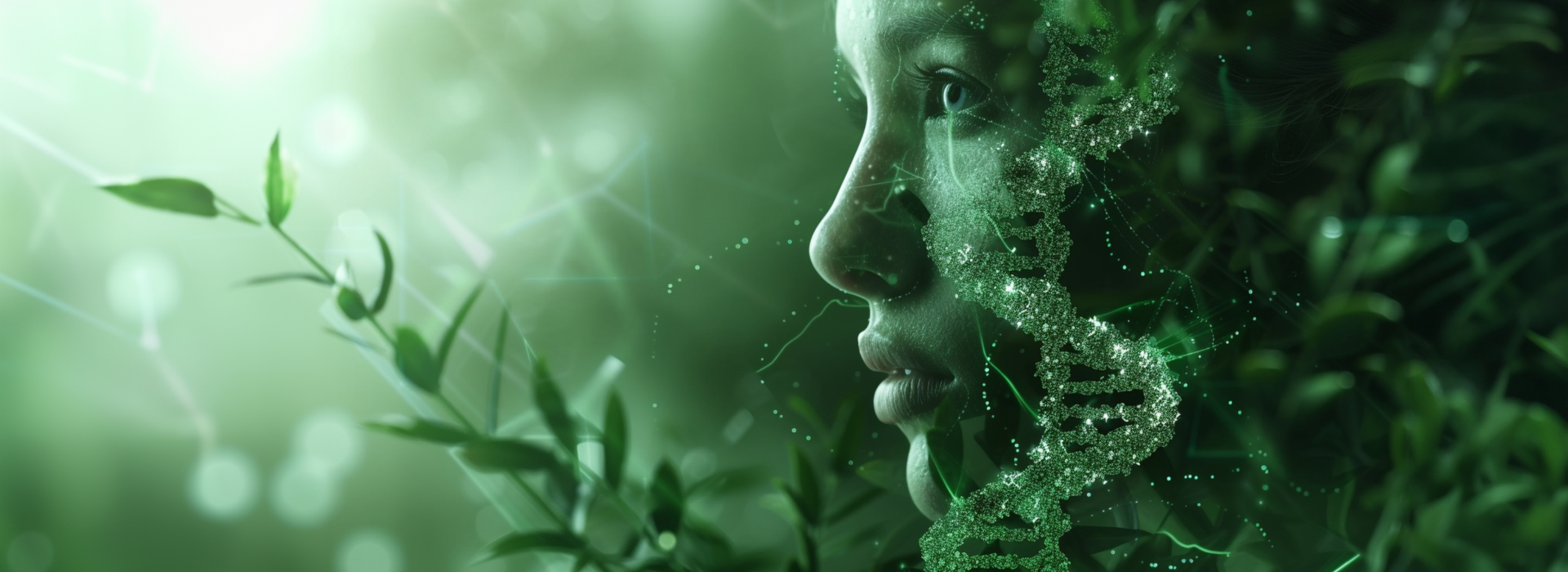
Challenges of using AI PaaS
There are two key disadvantages of using AI PaaS tools and services, both connected to the way these services are delivered. Let’s look closer:
- Limited customization options. One of the key issues regarding the use of AI PaaS is that you don’t have full control over it, as the range of customization options is usually limited. If your solution requires non-standard approaches, you may need to look for an AI vendor who allows full or at least extensive customization of pretrained models.
- Security compliance concerns. In order to get highly accurate results, ML models require lots of data. However, when using PaaS, you become dependent on your service provider’s security controls. Therefore, when choosing a provider, you need to make sure that all data is stored and transferred securely, especially if you store it in a public cloud.
Next, let’s look at the four providers who are closest to offering a full-scale AI PaaS so far.
Top 4 AI PaaS providers
The leading CSPs offering AI services are Amazon, IBM, Microsoft, and Google. All of them offer not only ready-to-go AI solutions for businesses but also tools for developers and data scientists, thus being both AIaaS and AI PaaS providers.
Let’s take a closer look at each provider and explore the value of their AI-related services.
AWS AI services
Amazon Web Services (AWS) provides a wide variety of dedicated tools and features for working with artificial intelligence. As an AIaaS provider, AWS offers 12 services, including text-to-speech capabilities, chatbots, automated code review, image and audio analysis, fraud detection, and demand forecasting.
Apart from that, AWS can also be considered an AI PaaS provider, since they offer the following services for development teams:
Let’s briefly describe how the mentioned AI PaaS services can help AI development teams:
- Amazon Augmented AI allows you to implement human review of sensitive data and ML predictions to ensure the model’s continuous improvement.
- Amazon SageMaker is a platform for building, training, and deploying ML models with fully managed infrastructure, tools, and workflows.
- Amazon SageMaker Data Labeling helps you create high-quality datasets for training ML models by identifying raw data and adding informative labels.
- Amazon SageMaker Neo automatically optimizes the speed of ML models for inference with no loss in accuracy, both for edge devices and in the cloud.
- AWS Deep Learning AMIs provide environments to help developers quickly build and scale deep learning (DL) applications.
- AWS Deep Learning Containers are Docker images with pre-installed DL frameworks. This service allows developers to deploy ML environments fast and easily without the need to build and optimize them from scratch.
Related project
Building AI Text Processing Modules for a Content Management Platform
Explore how our AI text processing modules empowered a global content management provider to enhance their platform and delight users worldwide. Discover the impact of AI-driven translation, generation, and formatting on user experience and platform efficiency.
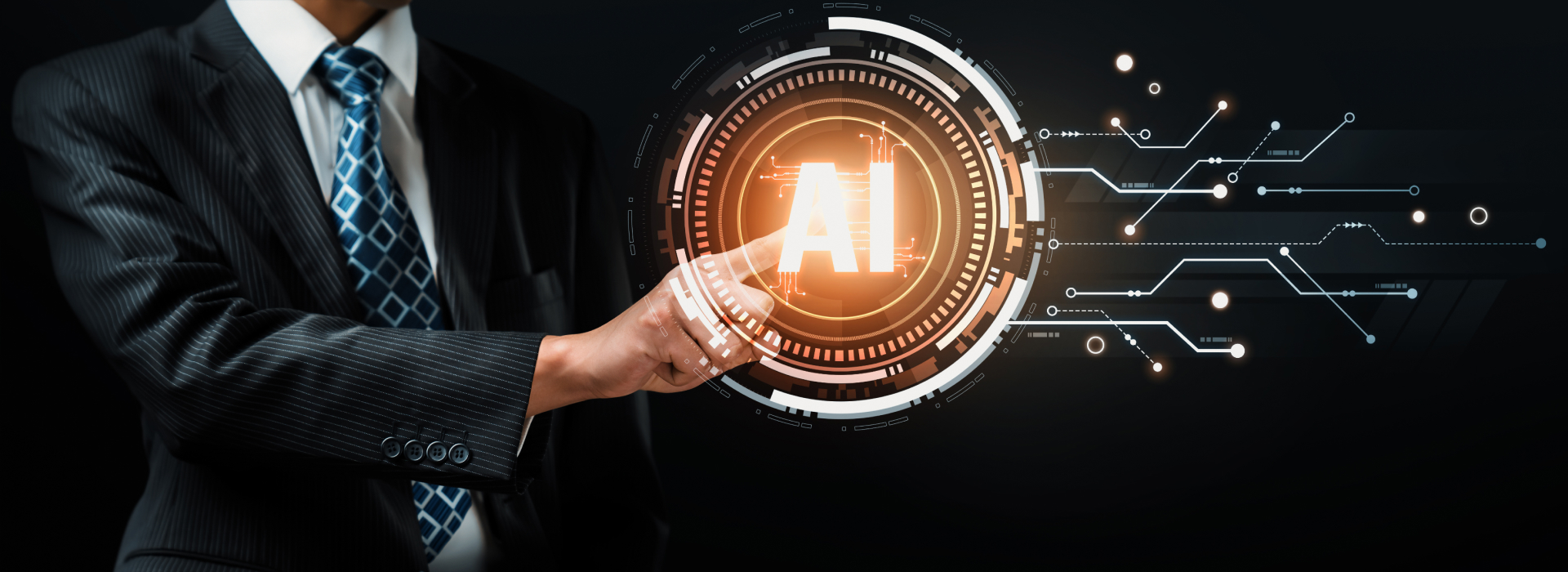
Vertex AI
Vertex AI, delivered by Google Cloud, is a unified artificial intelligence platform that offers pretrained and custom tools to help developers build, deploy, and scale ML models.
The key functionalities of this AI PaaS are:
- Vertex AI Data Labeling helps to annotate training data.
- Vertex AI Feature Store is a fully managed repository that allows developers to serve, share, and reuse ML features.
- Vertex AI Experiments allows you to track, analyze, and discover ML experiments for faster model selection.
- Vertex AI TensorBoard helps you visualize ML experiments.
- Vertex AI Pipelines simplifies the MLOps process by streamlining the building and running of ML pipelines.
- Vertex AI Training provides fully managed training services for ML models.
- Vertex Explainable AI offers detailed model evaluation metrics and feature attributions, and it shows how important each input feature is to your prediction.
Apart from Vertex AI, Google Cloud also offers Deep Learning VM Image. It’s a fully configured environment for building deep learning projects that supports all popular AI frameworks, including TensorFlow and PyTorch.
IBM Watson
IBM Watson is a suite of both general-purpose and industry-specific AI services, applications, and tools. IBM provides a wide variety of ready-to-go AI-driven solutions for businesses, including chatbots, natural language processing, virtual assistants, and detection of emotional and language tones in written text.
IBM Watson also provides a large selection of tools and services for preparing data and building and training ML models, including the following:
- IBM Watson Machine Learning is a service for developers and data scientists to work on creating and training ML models.
- IBM Watson Studio is a platform that helps data scientists, developers, and analysts to build, run, manage, and scale AI models in any cloud.
- IBM Watson OpenScale is a service for analyzing AI models, understanding how they make decisions, detecting and mitigating bias, and increasing the accuracy of predictions.
Read also
How to Develop Smart Chatbots Using Python: Examples of Developing AI- and ML-Driven Chatbots
Examine how AI chatbots can improve customer communication, reduce support costs, and automate routine business tasks. Use our practical example of Python-based chatbot development with GODEL and ChatterBox as a basis for your own chatbot development.
Azure AI
Azure AI is a comprehensive set of AI services designed to help developers and data scientists build and deploy their own AI solutions. This platform offers access to high-quality vision, speech, language, and decision-making AI models through simple API calls.
- Azure Applied AI Services help to accelerate development with built-in business logic and modernize business processes with task-specific AI models.
- Azure Cognitive Services is a family of customizable cognitive APIs for vision, speech, language, and decision-making.
- Azure Machine Learning is an end-to-end platform for building, training, and deploying ML models.
- AI infrastructure provides an opportunity for developers to build and train AI models within large-scale infrastructure.
Apart from that, Microsoft Azure also offers:
- Knowledge mining — a set of AI platform services for extracting insights from your content and turning forms into usable data
- OpenAI Service — a selection of fine-tuned language models for different use cases from summarization to content and code generation
- Azure Databricks — a platform for big data analytics and AI solution development
What to consider when choosing an AI PaaS service?
As you can see, while these AI service vendors offer similar sets of AI capabilities, there are some nuances and limitations. Therefore, your choice of an AI PaaS vendor should depend solely on the needs of your products.
Here are some final thoughts on what to pay attention to:
- Data quality – No matter what AI service provider you work with, the efficiency of AI features fully depends on the quality of the data processed. Make sure to put enough effort into the data preparation stage and use reputable databases with quality content. Consider using AI-based labeling services if you don’t have enough resources to label data manually. But make sure to check the results afterwards, however, since such tools can show different accuracies depending on the type and quality of input data.
- Compatibility of technologies – Pay special attention to the set of tools, services, frameworks, and programming languages supported by a particular AI PaaS. The more frameworks and tools that match your team’s experience and project needs, the easier it will be for your development team to work with the platform.
- API availability – Many AI service providers offer APIs for integrating AI capabilities in your application. Using APIs, you can introduce new AI functionalities into your software faster and with less effort. Make sure to check AI PaaS offerings for such solutions before starting to develop custom APIs.
Conclusion
AI PaaS empowers businesses with a variety of helpful AI features and capabilities, which in turn can accelerate and simplify the development of intelligent applications. Such platforms also provide collaboration opportunities for developers, data engineers, and business analysts, which is important for the growth and evolution of artificial intelligence technology.
However, working with AI PaaS can hide some pitfalls like lack of customization opportunities and concerns regarding security compliance. So make sure to research how AI providers handle data and what customization opportunities they offer before choosing one to work with.
At Apriorit, we have an expert AI development team with experience creating efficient AI-powered solutions for healthcare, cybersecurity, automotive, and other industries. We’ll be happy to help you conduct proper research, choose the most suitable AI PaaS provider, deliver a robust product, and assist you with testing artificial intelligence systems.
Let’s develop extraordinary AI solutions together!
Leverage Apriorit’s expertise in custom AI development to quickly get yourself efficient, secure, and tailored software.