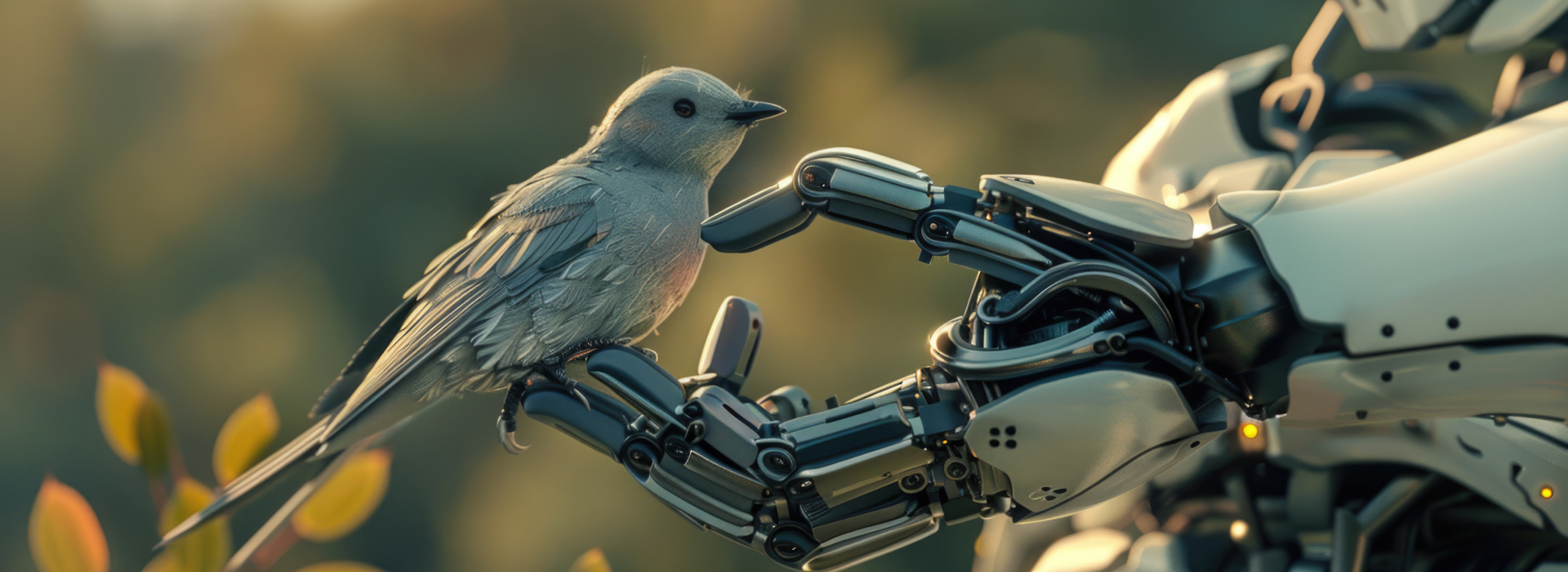
AI for Demand Forecasting: Benefits, Use Cases, and Implementation Tips
AI for demand forecasting: Explore business benefits, use cases, and Apriorit’s tips on how to create effective AI-based solutions.
Meet Apriorit Team at Web Summit Vancouver 2025
Vancouver Convention Centre, Canada
|
May 27-30
AI for demand forecasting: Explore business benefits, use cases, and Apriorit’s tips on how to create effective AI-based solutions.
Get your vehicle software closer to ISO 26262 compliance with key information on ensuring automotive functional safety.
Learn how to develop a virtual disk driver for Windows that ensures secure data storage, seamless integration, and high system performance.
Looking for the best reverse engineering tools? Read our review of the top reverse engineering programs for different operating systems.
Explore the latest RSA Conference 2025 cybersecurity trends and learn how Apriorit can help organizations adapt to threats.
Learn how to reverse engineer software on Windows legally using proven techniques, essential tools, and insights from Apriorit experts.
Explore key benefits and use cases for AI in FinTech to make strategic decisions when enhancing your FinTech software solutions.
Enhance cybersecurity data visualization with advanced analytics, real-time threat insights, and intuitive visual models for faster threat detection.
Explore top FinTech cybersecurity risks and discover best practices to protect your app from threats, breaches, and compliance issues.
Discover how to build a secure AI chatbot with key risks, best practices, and strategies to protect data, users, and business systems.
Tell us about
your project
...And our team will:
Do not have any specific task for us in mind but our skills seem interesting? Get a quick Apriorit intro to better understand our team capabilities.